State of natural language processing in surgery: Part 2
By: Cynthia Saver, MS, RN
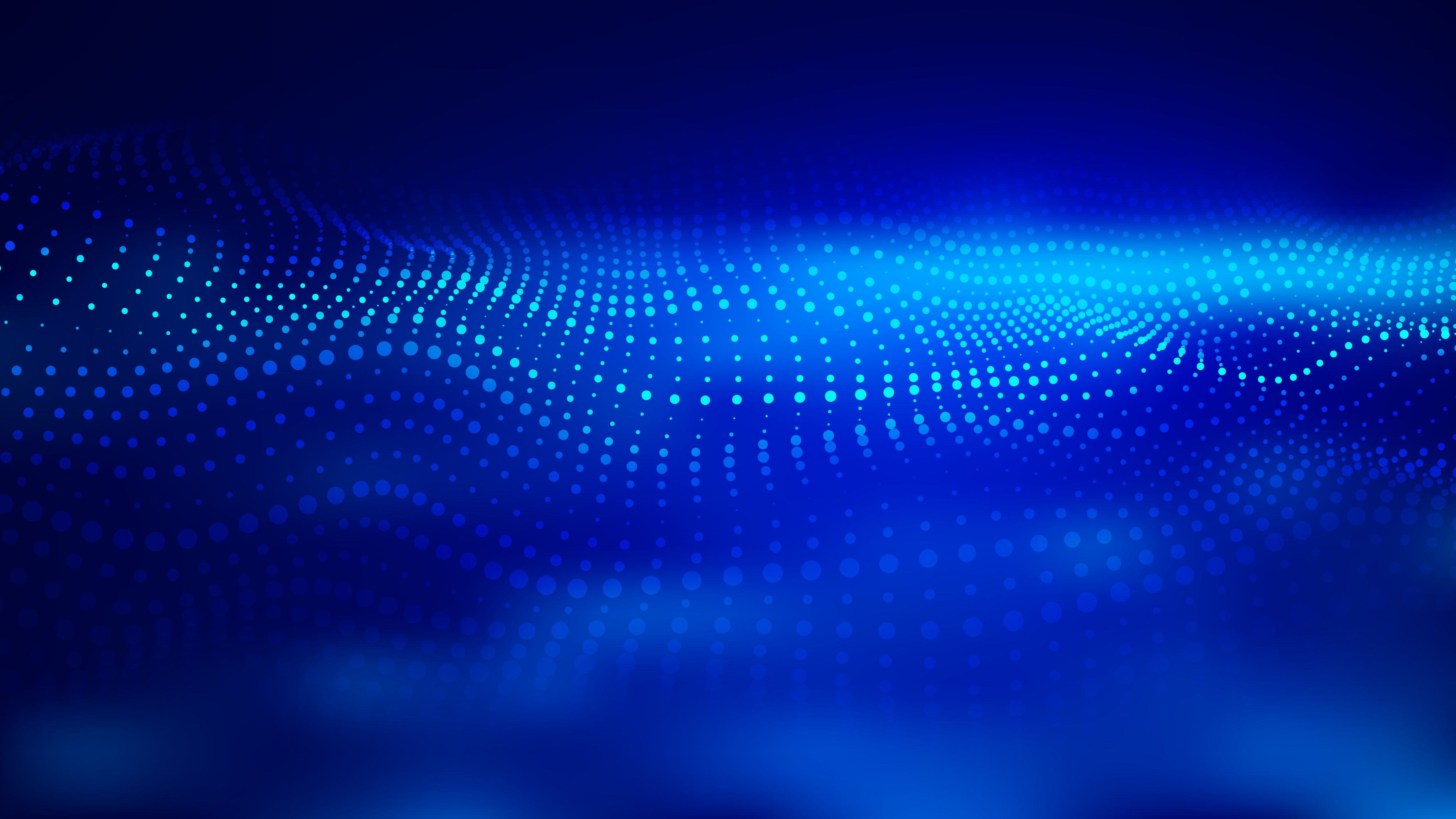
Natural language processing (NLP) has the potential to make clinicians’ work easier, help meet organizational goals, and promote quality patient care.
“This technology is something that can help us as healthcare stakeholders—whether nurses, administrators, or surgeons—to access a lot of the health information and data that are being generated every day,” says John Fischer, MD, director of the clinical research program, division of plastic surgery, and associate professor of surgery at the Hospital of the University of Pennsylvania in Philadelphia. “I see it as a very useful tool in helping us to make sense of and label a vast array of health information so we can do a better job of taking care of our patients.” Dr Fischer is also a co-author of a systematic review and meta-analysis on the use of NLP in surgery.
Part 1 of this two-part series explored some of the uses of NLP in surgery. Part 2 discusses the role of NLP in outcomes and general clinical research, as well as challenges of the technology and factors OR leaders should consider when purchasing products that use NLP (sidebar, Surgery-related uses of NLP).
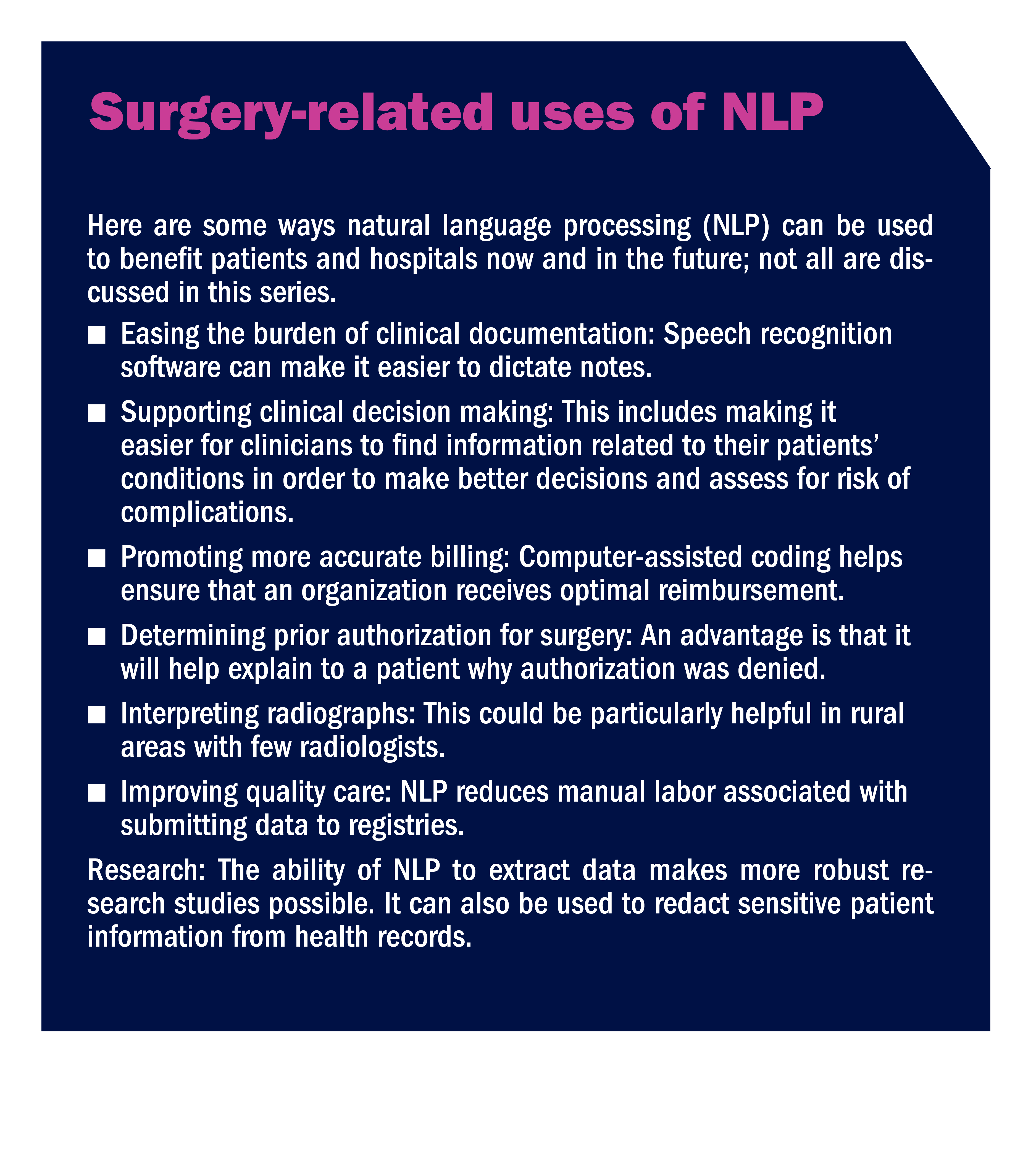
Outcomes
Many researchers are investigating the role of artificial intelligence (AI) that includes NLP to predict outcomes. For instance, the SORG Orthopaedic Research Group has explored the ability of machine learning, aided by NLP-developed algorithms, to detect or predict adverse outcomes. Examples include an algorithm for predicting 90-day and 1-year mortality in patients with cancer who underwent surgery for spinal metastasis. This type of information can help inform treatment discussions between clinicians and patients.
The SORG group also developed an algorithm for predicting which patients undergoing elective inpatient surgery for lumbar disc herniation or degeneration would not be able to go home after hospital discharge. This could help alert clinicians to the need for more interventions to prevent non-home discharges. Another of the group’s studies found that an NLP algorithm was effective for predicting 90-day readmission after elective lumbar spinal fusion surgery; the most useful notes for this were discharge summary notes.
Other examples of NLP-related outcomes studies include those by Shi et al, Karhade et al, and Bovonratwet et al (see reference list).
Clinical research
Advancing clinical practice depends on research, and having more data to analyze helps make that research more robust. “There is a rapid growth of using NLP in healthcare research as a tool to label data,” Dr Fischer says.
NLP enables the creation of large databases of information that researchers can draw on. One example is the National COVID Cohort Collaborative, which has more than 20 billion rows of data from more than 260 sites that send the collaborative their electronic health record (EHR) data.
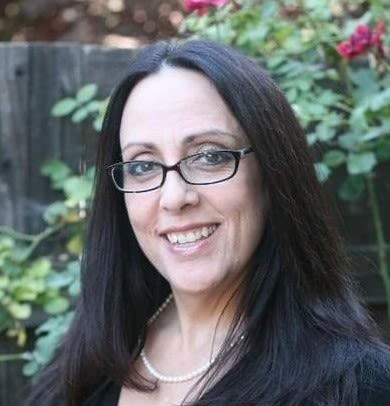
Davera Gabriel, RN
Davera Gabriel, RN
“NLP is being used to extract structured data from unstructured components,” says Davera Gabriel, RN, director for terminology management in biomedical informatics and data science at Johns Hopkins University in Baltimore, who works on the project. Sources like notes from nurses and social workers contain key information that can affect recovery from COVID-19, including determinants of health such as food insecurity.
The higher volume of data that NLP can gain from EHRs also can help answer clinical questions in cases where the volume of available data is sufficient for statistical power.
Challenges of NLP
As with any technology, NLP comes with a set of challenges that may impact its usefulness.
Bias. Biases in AI are a concern because they can be built into the data used to develop AI. Less is known about biases specific to NLP, but it likely has similar issues.
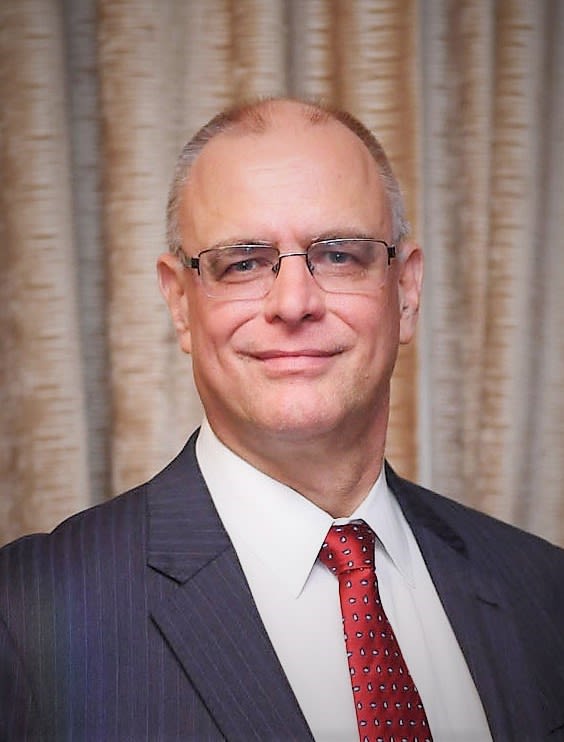
J. Marc Overhage, MD, PhD
Dimitrios Stefanidis, MD, PhD
“The model is only as good as the information on which it’s trained,” Dr Fischer says. “If a population is underrepresented or misrepresented in the training set, the algorithm won’t perform well on validation.” Providers’ conscious or unconscious attitudes may influence how information is entered into the EHR, resulting in bias, says J. Marc Overhage, MD, PhD, chief medical informatics lead for Elevance Health. Bias could occur in several areas, including sex, race, and socioeconomic status. “Whatever biases live in the data will live on in the algorithm,” Dr Overhage says.
“The issue of training [a machine] on an existing data set is that data set currently contains all the presuppositions that led to that circumstance,” says Yaa Kumah-Crystal, MD, MPH, clinical director for health IT in the biomedical informatics department at Vanderbilt University Medical Center in Nashville, Tennessee. “It’s not just ‘set it and forget it’: You have to continue to evaluate it so the model can continue to learn.”
For example, a 2021 study by Wong and colleagues found that the sepsis prediction model used in Epic’s EHR did not identify two-thirds of patients with sepsis. In its response to the study, Epic noted: “Each health system needs to set thresholds to balance false negatives against false positives for each type of user. When set to reduce false positives, it may miss some patients who will become septic. If set to reduce false negatives, it will catch more septic patients; however, it will require extra work from the health system because it will also catch some patients who are deteriorating, but not becoming septic.” This points to the need to validate whether a model used in one organization is effective in another.
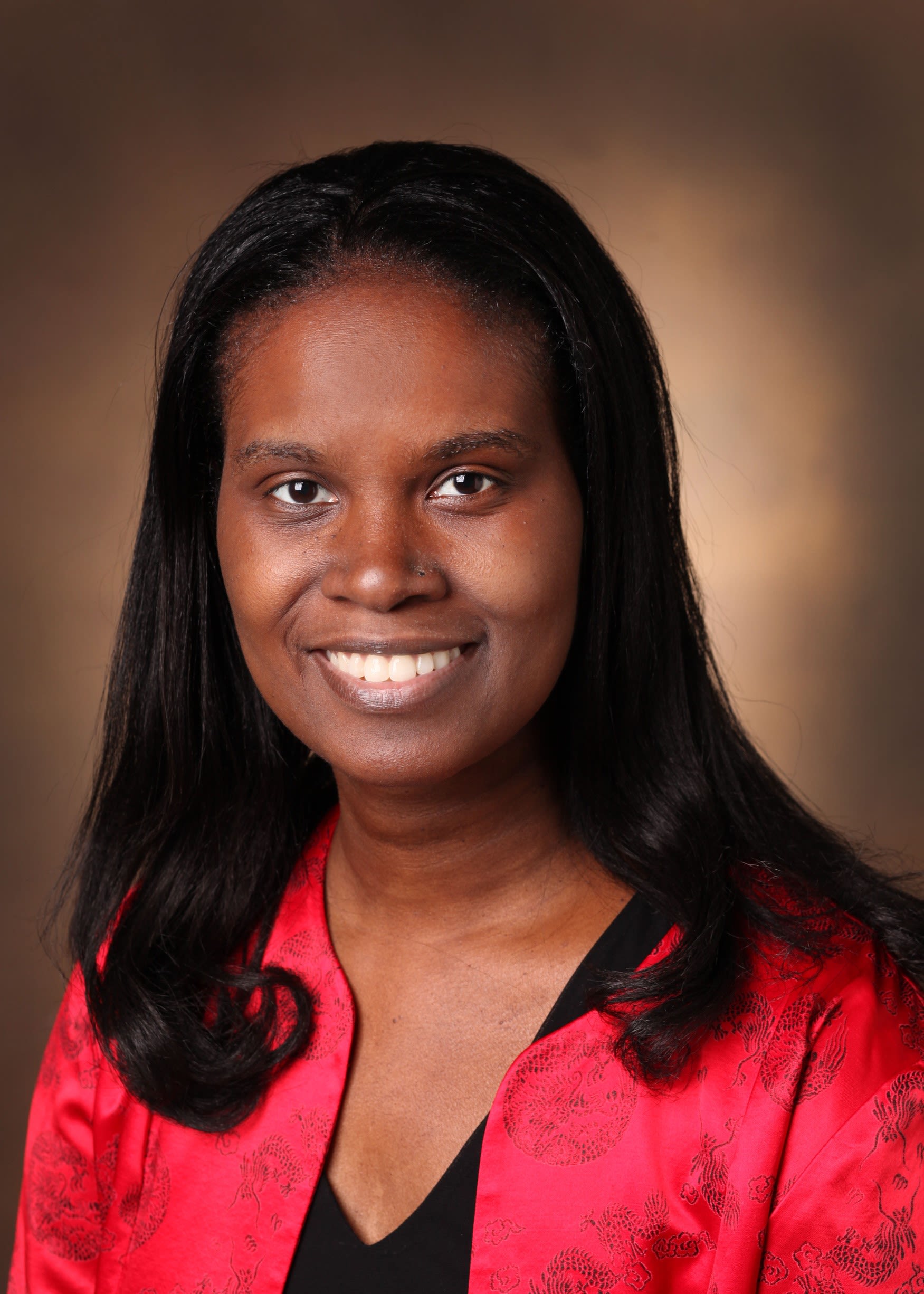
Yaa Kumah-Crystal, MD, MPH
Dimitrios Stefanidis, MD, PhD
Dr Kumah-Crystal, who also is a pediatric endocrinologist who works on improving the usability of EHR through NLP, says another way to address bias is to have an advisory panel with representation from clinicians, ethicists, social scientists, and patients who can help determine the types of questions that a computer should be asking. For example, social determinants of health such as homelessness should be incorporated if they are representative of the patient population being treated. “IT people are data optimistic—we see all the good things that can come from it,” she says. “But it’s important to have other people at the table who might have a different lens. [For example,] patients can give you different insights that you might not think about because you are walking a different path.”
Stigmatizing language plays a role in bias. For example, a nurse might write, ‘patient claims they stopped smoking a month before surgery but smoke odor noted on clothes,’ with ‘claim’ implying the nurse does not believe the patient. However, the odor might be because the patient lives with someone who smokes.
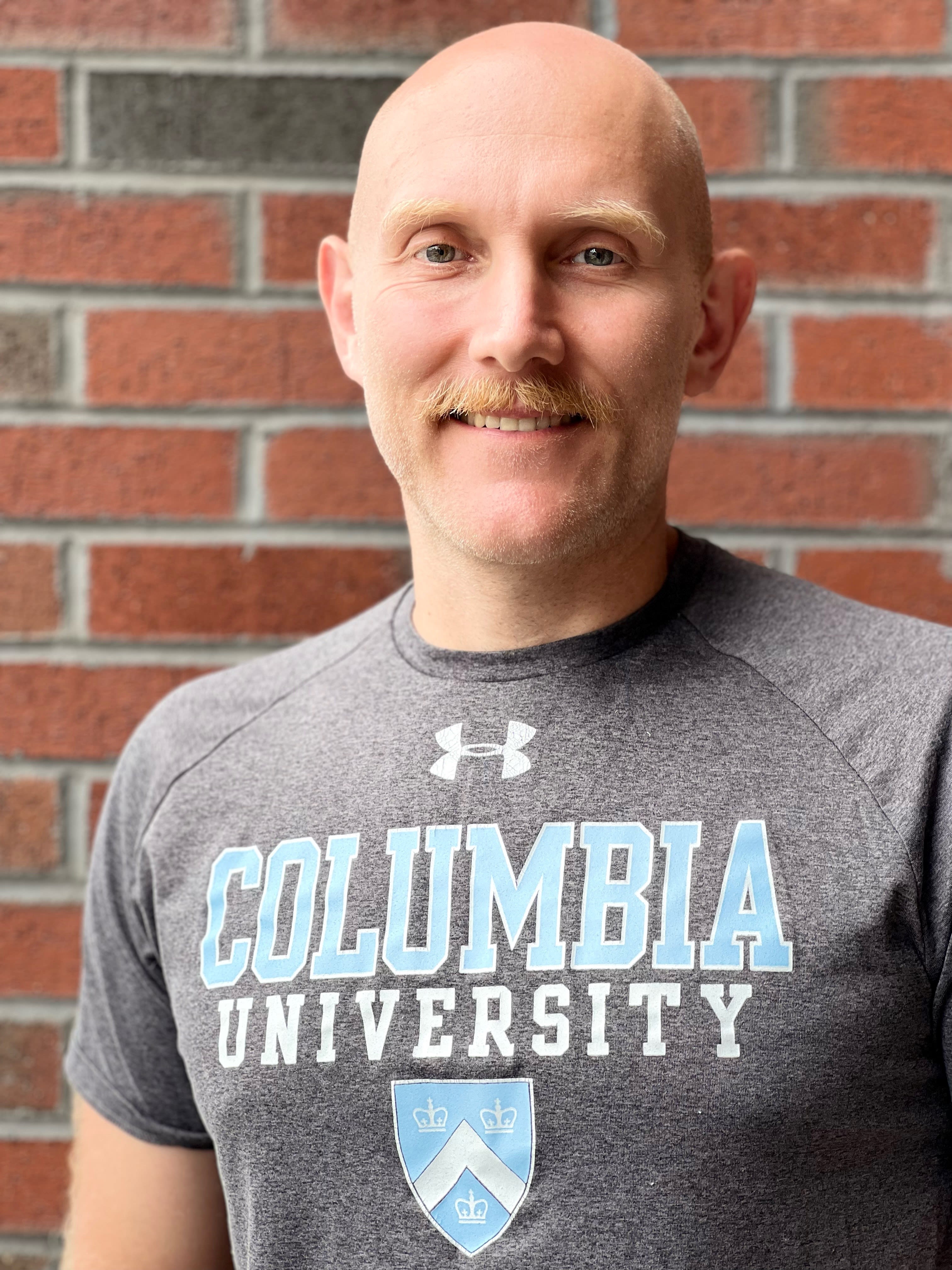
Maxim Topaz, PhD, MA, RN
Dimitrios Stefanidis, MD, PhD
“Biases are propagated through stigmatizing language,” says Maxim Topaz, PhD, MA, RN, the Elizabeth Standish Gill associate professor of nursing at Columbia University Medical Center and Columbia University Data Science Institute in New York. Topaz adds that this type of language is often used with minority communities. He is working on a way to use NLP to alert clinicians to the use of stigmatizing language so they can avoid it. This is particularly important given that clinicians are required to share notes with patients when requested.
Cost. Basic NLP voice recognition is inexpensive and widely available, but the power of NLP lies in its ability to analyze and learn. Those capabilities require neural networks and deep learning techniques that are advanced technologies, and, therefore, expensive.
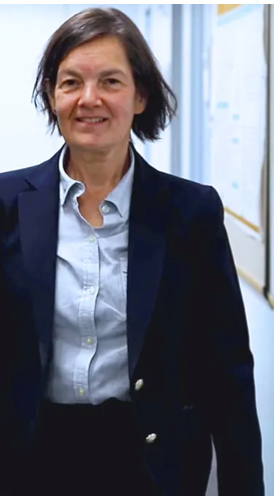
Dina Demner-Fushman, MD, PhD
Dina Demner-Fushman, MD, PhD
Privacy. Privacy is a concern, particularly when it comes to the large-scale use of EHRs that are needed by researchers to provide optimal results. “Hospitals are not able to share much information because of HIPAA restrictions, and there are few places where researchers can buy data for research,” says Dina Demner-Fushman, MD, PhD, an investigator at the National Library of Medicine in Bethesda, Maryland. She is also the chair of the American Medical Informatics Association NLP work group; her research includes the use of NLP to extract and use data for clinical decision support and education.
When data are shared for research purposes, steps must be taken to de-identify it. At the National COVID Cohort Collaborative, Gabriel says the team uses a variety of methods to ensure HIPAA compliance. One method, for example, is to use only 3-digit ZIP codes and to randomly shift dates in the record while still maintaining the sequence of events.
Technical and terminology barriers. The number of commercial products using NLP is increasing but still limited, with more information needed about their capabilities, Topaz says. “Not every organization has the IT ability to tweak the model,” he adds.
Another technical issue is that risk prediction models need to be specific to an organization because the terminology clinicians use and patient populations can vary widely between geographic locations and clinical settings. Dr Fischer notes that when dialects and terminology vary because of geographic region, it may influence how effectively an NLP-generated algorithm functions.
For example, what works for clinicians in Philadelphia may not work for clinicians in San Francisco, where there are different populations of people who use different terminology. “It really speaks to the importance of the NLP algorithm being a living thing,” he says. “Tools need to be tested to ensure performance is maintained in a new geographic setting.”
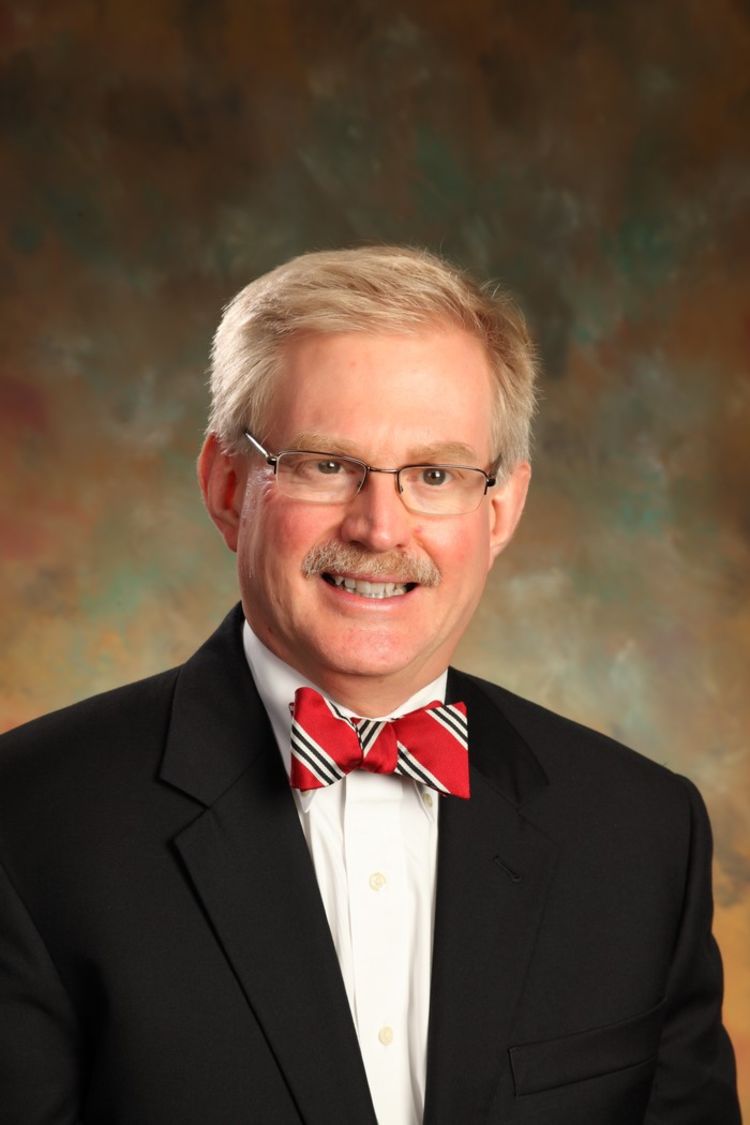
Stephen Morgan, MD
Dimitrios Stefanidis, MD, PhD
A new way of thinking. NLP enables predictive analytics, which requires a new way of thinking for clinicians, says Stephen Morgan, MD, senior vice president and chief medical information officer for Carilion Clinic in Roanoke, Virginia. “It has been more of a learning curve for clinicians to integrate these tools and data insights into their practice than we anticipated.”
Jennifer Martin, DNP, RN, NEA-BC, senior director of clinical informatics at Carilion, advises clinicians to consider NLP and other forms of AI as partners in delivering care. “It helps point out a little sooner than our natural brain process that there is an issue, but nurses and physicians still have to understand how to incorporate it into their practice,” she says. “The machine will put the data together logically, but it may not be accurate.”
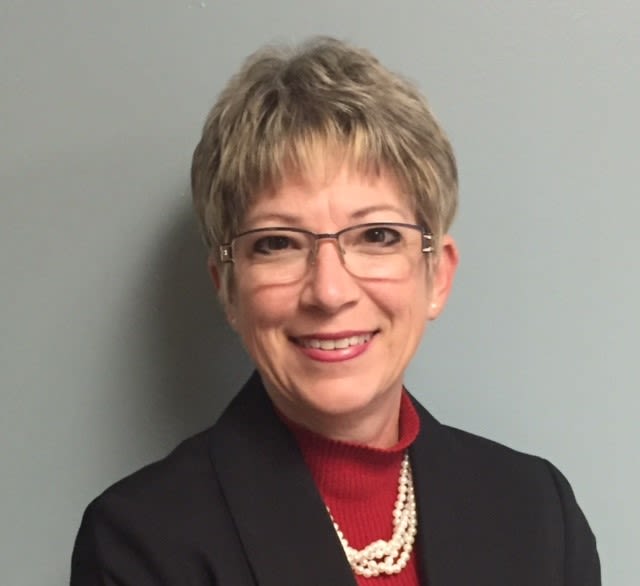
Jennifer Martin, DNP, RN, NEA-BC
Jennifer Martin, DNP, RN, NEA-BC
For example, a nurse may not have documented an action that would impact what the machine is concluding. “You still have to use critical thinking and look at your patient,” says Martin, who uses the analogy of an ECG monitor alarming because of a flat line, but the patient is fine, indicating a mechanical, not physiological, problem. However, she adds, “[Information obtained through NLP and AI] can help us [clinicians] react faster and bring information together we have not considered.”
Dr Demner-Fushman says dashboards that incorporate results from NLP tools need to aggregate the results for clinicians, who do not have the time to do it themselves. She worked with a team to create a dashboard for interdisciplinary teams that included patient information and resources such as practice guidelines and current information on the patient’s diagnosis and medications. When Dr Demner-Fushman explored why usage did not grow as expected, most clinicians said they did not have time to read the information and wanted more of a summary. “We’re working on trying to accomplish that,” she says.
Making a purchase
What should OR leaders think about when evaluating NLP tools for purchase?
Experts interviewed for this article cite these main considerations:
- usefulness of the technology
- number of people who will use it
- ease of use
- integration of the technology into the workflow.
It is important not to oversell what the technology can do, but rather, Dr Overhage says, find the best intersection between the clinician and the technology. He cites the example of Digital Nurse, which uses NLP to help clinicians identify where in the EHR they need to focus their attention. NLP organizes information and highlights key points so clinicians do not have to read through massive amounts of information. “It’s the person and the technology together,” Dr Overhage says. “It’s better than the person can do on their own, but it’s not asking the technology to do too much.” Digital Nurse is being used by Elevance’s care managers.
“Trust, but verify,” Dr Morgan says, referring to the need to ensure the NLP processing is accurate. “Most vendors will estimate the accuracy of their product, but given the complexity of the data and the algorithms used, we try to verify as much as we can to ensure data quality.” A simple example is ensuring the program correctly differentiates between a patient having cancer and a family history of cancer.
Experts agree that, as with any technology purchase, an organization should talk with previous purchasers to gain their insights.
Once the technology is purchased, clinicians must be trained on how to use it both practically (how to enter and access information) and intellectually (how to integrate the information into practice). The intellectual education can be challenging, particularly with predictive models. “As we’re developing these advanced analytic models, there is still a lot to learn as to how to best present this to clinicians,” Dr Morgan says. Clinicians should document in a timely manner so the system can be most effective.
Martin cites the Rothman Index, which tracks 26 variables related to illness severity, as an example of how a tool that uses machine learning can be helpful to organizations, clinicians, and patients. A Rothman Index score that indicates a patient is deteriorating triggers an alert on a supervisor’s mobile device. The supervisor then checks the EHR and goes to the unit to investigate. Although in many cases the nurses are already taking action (but have not yet documented their steps), the index is also used to open a discussion about whether a planned transfer out of the ICU should be reconsidered.
The Rothman Index has another benefit, Martin says: It can boost the confidence of inexperienced nurses. “It gives them one more piece of information to support their feeling that something might be happening with the patient.”
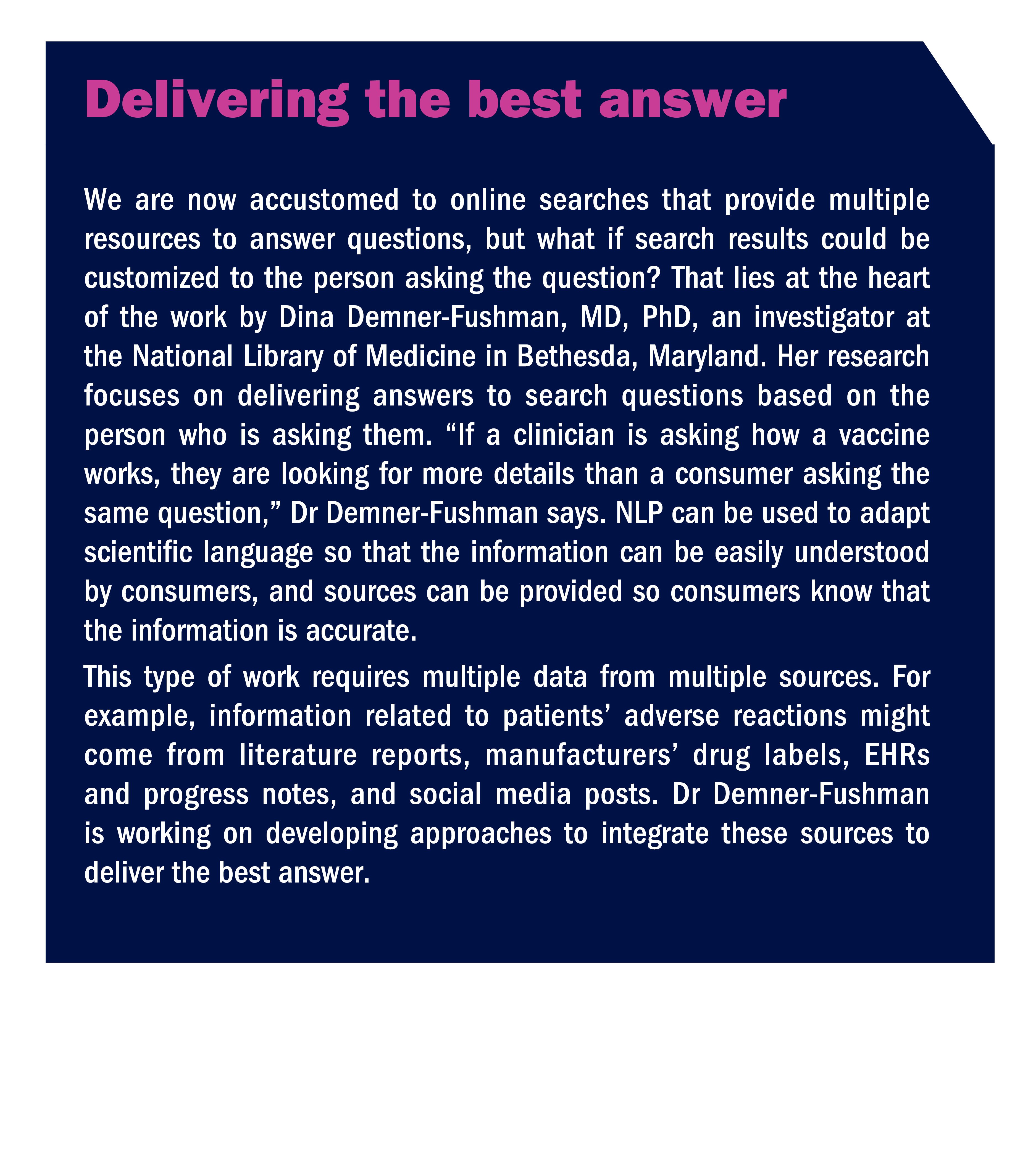
Future potential
NLP has the potential to put nursing notes front and center of patient care. “Research has shown us that nursing notes are valuable for optimal patient care, so we need to make better use of them,” Topaz says, adding that too often notes go unread. “We need [AI] like [NLP] to make use of that important source of information.” He notes that being able to apply NLP to the everyday workflow will make the clinician’s life easier.
In the OR of the future, clinicians will be able to easily search the EHR for needed information. “It’s surprising how difficult it is to search the EHR compared to a Google search,” Topaz says. Dr Demner-Fushman says the ultimate goal is a paperless hospital: “The moment the patient comes in, the computers do everything to support clinicians: That’s what we are working towards.”
In such a hospital, clinicians will be able to speak a question and receive “snippets” of information that is most pertinent to the question. NLP will help computers better understand the question being asked, Dr Demner-Fushman says.
For example, instead of simply asking how to treat a certain disease, the clinician could ask how to treat it in a patient of a certain age with certain comorbidities. “Patients are complicated, so the questions asked are often complicated,” says Dr Demner-Fushman, who is also exploring how NLP can help consumers receive better answers to their health-related questions (sidebar, Delivering the best answer).
“With power comes great responsibility,” Dr Fischer says. “This tool has power and needs to be used responsibly. It could be used poorly and potentially have bad consequences for healthcare, or, if used thoughtfully and appropriately, it could transform the way we do research and the way we take care of patients.”
References
Bovonratwet P, Shen T S, Islam W, et al. Natural language processing of patient-experience comments after primary total knee arthroplasty. J Arthroplasty. 2021;36(3):927-934.
Karhade A V, Bongers M E R, Groot O Q, et al. Can natural language processing provide accurate, automated reporting of wound infection requiring reoperation after lumbar discectomy? Spine J. 2020;20(10):1602-1609.
Karhade A V, Bongers M E R, Groot O Q, et al. Development of machine learning and natural language processing algorithms for preoperative prediction and automated identification of intraoperative vascular injury in anterior lumbar spine surgery. Spine J. 2021;21(10):1635-1642.
Karhade A V, Lavoie-Gagne O, Agaronnik N, et al. Natural language processing for prediction of readmission in posterior lumbar fusion patients: Which free-text notes have the most utility? Spine J. 2022;22(2):272-277.
Mellia J A, Basta M N, Toyoda Y, et al. Natural language processing in surgery: A systematic review and meta-analysis. Ann Surg. 2021;273(5):900-908.
Shi J, Hurdle J F, Johnson S A, et al. Natural language processing for the surveillance of postoperative venous thromboembolism. Surgery. 2021;170(4):1175-1182.
SORG Orthopaedic Research Group. Predictive algorithms.
Wong A, Otles E, Donnelly J P, et al. External validation of a widely implemented proprietary sepsis prediction model in hospitalized patients. JAMA Intern Med. 2021;181(8):1065-1070.